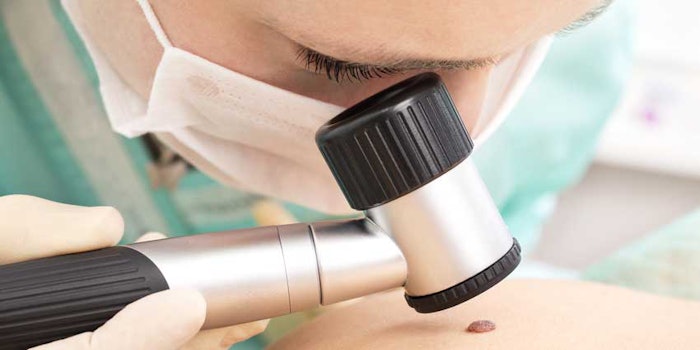
Melanoma can be a tricky type of cancer to detect due to the moles resembling non cancerous ones. However, researchers at the Rockefeller University developed an automated technology—combining images with digital analysis and machine learning—to detect melanoma at its early stages.
"There is a real need for standardization across the field of dermatology in how melanomas are evaluated," said James Krueger, D. Martin Carter professor in Clinical Investigation and head of the Laboratory of Investigative Dermatology. "Detection through screening saves lives, but is very challenging visually and even when a suspicious lesion is extracted and biopsied, it is confirmed to be melanoma in only about 10% of cases."
The Q-score’s Significance
The new method to examine these moles takes images of lesions and processes them through a series of computer programs, which then churn out information about the number of colors present in a growth and other quantitative data.
What helps detect whether the growth is cancerous is the overall risk score, which is also known as a Q-score. According to a recent study published in Experimental Dermatology, the Q-score tool was evaluated and its efficiency yields 98% sensitivity, which helps physicians correctly identify early melanomas on the skin.
The capability of the test to correctly diagnose normal moles was 36%, which is similar to what expert dermatologists accomplished in performing visual exams of suspicious moles under a microscope.
"The success of the Q-score in predicting melanoma is a marked improvement over competing technologies," said Daniel Gareau, first author of the report and instructor in clinical investigation in the Krueger laboratory.
Discovering the Melanoma Moles
Image processing programs were supplies with 60 photos of cancerous melanomas and 60 photos of non cancerous growths, which was how the Q-score tool was developed. The programs created imaging biomarkers to accurately count visual features of the growths.
Computational methods produced a set of quantitative metrics, which varied between the two image groups, helping identify what visual aspect of the lesion was most significant in terms of malignancy and giving each biomarker a malignancy rating.
The overall Q-score for each image was calculated at a value between zero and one after combining data from each biomarker. The higher the value number is, the higher probability a lesion has in being cancerous.
Another Perspective
Previous studies proved the most important biomarker for determining malignancy is the number of colors in a lesion.
Other biomarkers held significance when researchers looked in specific color channels, which could possibly be used to identify additional biomarkers and improve precision further.
"I think this technology could help detect the disease earlier, which could save lives, and avoid unnecessary biopsies too," said Gareau. "Our next steps are to evaluate this method in larger studies, and take a closer look at how we can use specific color wavelengths to reveal aspects of the lesions that may be invisible to the human eye, but could still be useful in diagnosis."
Source: MedicalXpress